DeepMass: A new machine learning method for the characterization of proteins
Exploring one of the first applications of deep learning to the field of mass spectrometry.
Proteins play a pivotal part in biology, from DNA replication to food digestion, and the function of proteins often plays a role in the development of disease. The presence and levels of certain proteins in our bodies, known as protein profiles, provide a direct, up-to-date window into the status of our health. Despite the importance of proteins, there are still major limitations in proteomics, or the study of protein profiles at a large scale. One challenge is that there are inadequate computational tools to interpret the data coming from experimental platforms that analyze proteins. To address this, our team—in collaboration with Jürgen Cox’s Computational Systems Biology research group at the Max Planck Institute of Biochemistry and Google—explored whether the application of machine learning could help. Today we are proud to announce that our joint manuscript "High-quality MS/MS spectrum prediction for data-dependent and data-independent acquisition data analysis" has been published in Nature Methods. With one of the first applications of deep learning in the field of mass spectrometry, we successfully demonstrated a more accurate method that significantly increases our ability to identify and characterize known biomarkers in a sample.
At Verily, we measure protein profiles using mass spectrometry in many clinical studies, including The Project Baseline Health Study, as part of our search for new biomarkers of disease. We also integrate protein signals with other biomolecular data like genomics and transcriptomics, as well as with device measurements and disease status, to find out how genetics and behavior affect protein profiles. To do this, we use an emerging method in protein mass spectrometry, dubbed data independent acquisition (DIA), that is able to identify and quantify proteins more accurately and precisely than previous methods. The DIA approach relies on experimentally determined spectral libraries for data interpretation, the development of which is time and resource intensive. As described in our presentation, our team of collaborators sought to develop a tool to generate spectral libraries by computation, which we’ve termed DeepMass. By using computation to develop spectral libraries, we hypothesized that we could more quickly develop the necessary reference material to interpret large datasets of protein profiles generated by mass spectrometry.
DeepMass is one of the first applications of deep learning to the field of mass spectrometry. In the released manuscript, we show that it is highly accurate at predicting peptide mass spectra: the cross-correlation coefficient between the actual and predicted spectra is 0.944, significantly better than the available state-of-the-art (0.871). This is just shy of the theoretical limit determined by technical variation (0.976), and crucial for discovery of biomarkers with small effect sizes. Moreover, in our first application to actual clinical data at Verily, we were able to expand the coverage of known biomarkers by more than two fold.
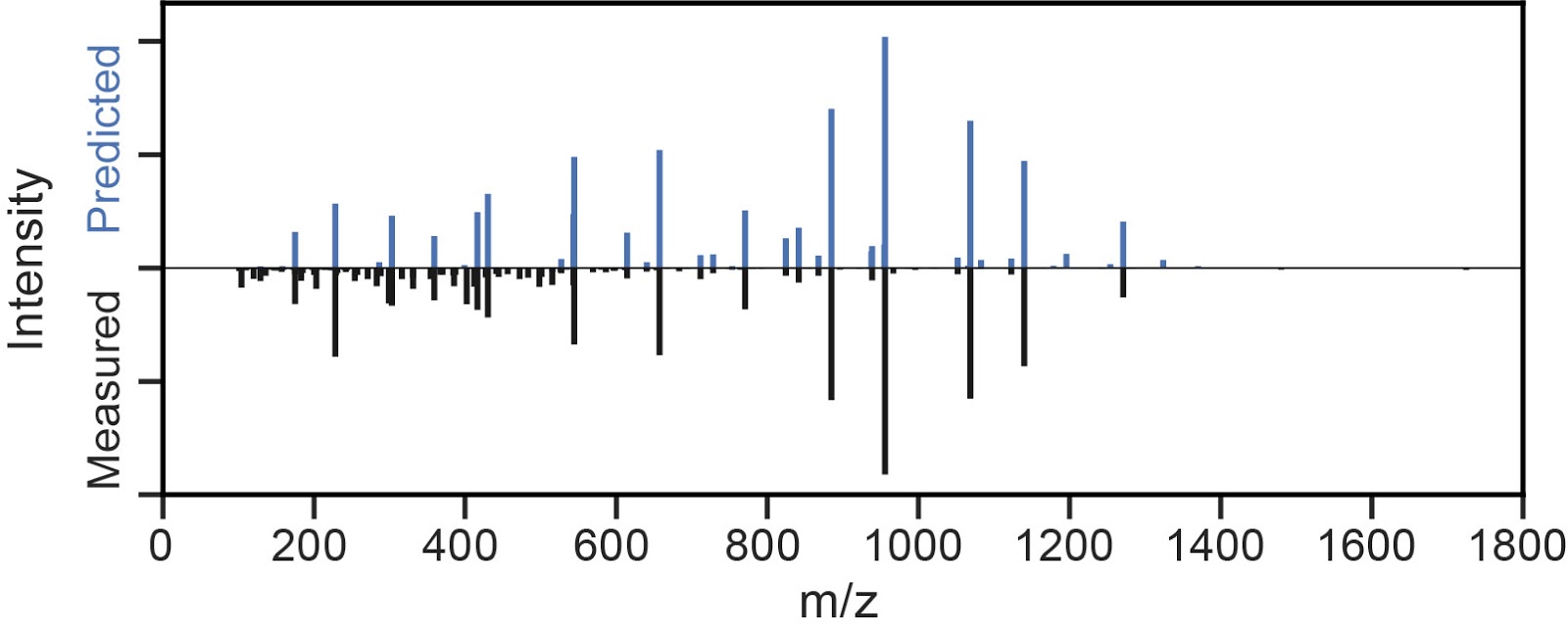
An example experimental peptide spectra [black, below] and its predicted peaks and intensities from DeepMass [blue, above].
Furthermore, we demonstrated that the utility of DeepMass-calculated spectral libraries is equivalent to the experimental ones. Additionally, we made a surprising discovery: When delving into the inner workings of our deep learning model, we not only saw that the model correctly learned known chemical rules that govern a peptide fragmentation, but also suggested some new ones.
Proteins are critical biomarkers of disease development and progression—the more we know about them and their relationship to specific diseases, the earlier and more precisely we can intervene. We hope that DeepMass, available on Google Cloud, will enable researchers to characterize disease-relevant protein profiles to build new diagnostic tools and therapeutics. We look forward to continuing the application of machine learning to proteomics, proteogenomics, and other fields, to further Verily’s mission of making health data useful so people can live healthier lives.